The big challenges that the textile industry currently faces are problems with the traceability of materials, material sorting at waste management centers, and overall end-of-life processing.
Material traceability in textiles has widely been an issue because there are innumerable amounts of raw material sources that one product could be manufactured from. When these textiles and garments are seen to be obsolete or soiled by the consumer, they are then disposed of and most likely sent to waste management facilities to be sorted, in which there are many limiting factors to the control of this process. Moreover, because of the poor sorting and disposal conditions of these textile goods, overall end-of-life processing is highly unregulated.
To combat these pressing issues, technologies such as radio frequency identification (RFID) tags, digital twin models, and deep learning artificial intelligence (AI) can be utilized to assist or create a new system of sustainable textile processing.
RFID
I’m currently a master’s student at Thomas Jefferson University studying textile technology and during our fall semester, we were given a semester-long project to come up with answers to the textile industry’s infamous traceability and sustainability issues.
This project allowed me to think of applicable solutions to these problems as well as permitted me to collaborate closely with big industry partners such as Waste Management of Philadelphia, Under Armour, and a digital ID company called EON.
My interdisciplinary team of peers came up with the idea of color-coded RFID tags sewn directly onto garments. The color of the tag would represent the material of the garment. For instance, blue would signify a natural fiber such as cotton, red would signify a synthetic fiber such as polyester, and possibly purple would represent a blend of both natural and synthetic fibers (such as a cotton/polyester blend). Other fibers that garments are typically made with would also receive a respective color and thus would have an overall visual aspect to sort these garments by fiber types.
Within the color-coded tag would be an RFID that contains information regarding the garment’s materials such as where the raw material was sourced, where it was manufactured, and how it should be processed when it reaches its end of life.
Having this RFID would allow companies such as Waste Management to scan these tags at their facility and sort these products to their respective end-of-life processing areas (such as recycling plants, landfills, burned as fuel, etc.).
That was our short-term goal.
Our long-term goal was to incorporate digital twin technology to track and monitor how successful the RFID tags were working in real-time throughout this system. Having digital twin modeling would allow us to closely monitor what is and what isn’t working within the supply chain as well as how the garment is being cared for from manufacturer to end-of-life processing.
These technologies and systems would ultimately work under a deep learning AI model to assist with material identification sorting, efficiency analytics, and working with industry partners to assist in the overall recycling or disposal of these textile products. This concept would seamlessly integrate color-coded RFID tags directly onto garments for two-factor identification at waste management facilities.
Traditional traceability methods often falter in the face of diverse materials used in textile production. Our solution employs a visual coding system that assigns unique colors to different materials, providing an efficient and intuitive sorting mechanism.
The tag attached to the garments would be made of recycled polyester for their high durability and colorfastness since they would be solution dyed for color-coding. Potential test methods that would be utilized to test for colorfastness of the resulting tags would be aligned with the American Society for Testing and Materials (ASTM) standards of the American Association of Textile Chemists and Colorists’ (AATCC): Test Method for Colorfastness to Laundering: Accelerated [AATCC TM61-2013e(2020)e2], Test Method for Colorfastness to Drycleaning [AATCC TM132-2004e3(2013)e3], and Test Method for Colorfastness to Perspiration [AATCC TM15-2021e].
Along with the visible aspect of identification, there would also be an RFID within the tag that could be scanned at processing facilities like Waste Management.
EON, the digital ID company we were collaborating with on this project had extensive expertise in RFID technology. From their feedback, we learned that these RFIDs were to be passive rather than active, as well as contain a circular antenna so they would be affordable for textile/clothing companies to implement and can be read in any orientation.
Active RFIDs were not chosen for this application due to their high price points and the potential hazard of causing electromagnetic interference with medical devices that can be affected by external radio frequencies such as pacemakers.
Each RFID would contain a wealth of information about the garment including details about the materials’ origins, the manufacturing process, and crucial instructions for end-of-life processing. The data would be associated with the RFID’s unique numerical code for each fiber or fiber blend type. By embedding this comprehensive information directly into the garment, we aimed to empower stakeholders at every stage of the supply chain with the knowledge needed to make informed, sustainable decisions.
This short-term goal centers around enhancing end-of-life processing and sorting at facilities like Waste Management. The RFID tags, meticulously color-coded and information-rich, enable swift and accurate sorting within these facilities, thus ensuring that garments are directed to their respective recycling processes, landfill areas, or alternative pathways based on their material composition.
This approach holds significant promise in reducing the environmental impact associated with textile waste. By collaborating with organizations like Waste Management, our project not only seeks to revolutionize traceability but also actively contributes to the broader movement towards circular economies and recycling initiatives. The efficient sorting facilitated by RFID tags is a tangible step towards minimizing the textile industry’s environmental footprint, aligning with the global commitment to sustainable waste management.
Digital twins
We aspire to usher in a new era of traceability and sustainability by incorporating digital twin technology into the fabric of the textile supply chain as well.
Digital twin technology is a virtual replica that mirrors the physical characteristics and behaviors of a system or process. In the context of our project, this translates into creating a dynamic, real-time system that monitors RFID tags throughout a garment’s lifecycle. By embracing digital twinning, we aim to achieve several critical objectives: optimizing supply chain efficiency, ensuring sustainable practices, and identifying opportunities for improvement.
Real-time monitoring allows for proactive decision-making within the supply chain, in which manufacturers, consumers, retailers, and even end-of-life processors gain insights into the movement and condition of garments, enabling them to streamline processes, reduce inefficiencies, and minimize waste.
With the integration of digital twin modeling, manufacturers can assess the environmental impact of their production processes, retailers can make informed decisions about stocking sustainable products, and consumers can be confident in the ethical and eco-friendly choices they make. This would also give end-of-life processors a sense of the volume and capacity that they could be expecting when these textiles are thrown away and end up in their respective domains. Continuous monitoring enables us to adapt and evolve our strategies in real-time, ensuring that our traceability and sustainability efforts remain at the forefront of industry standards.
Digital twin modeling also provides a comprehensive view of the entire supply chain. This bird’s-eye perspective allows us to identify bottlenecks, areas for improvement, and opportunities for innovation. Utilizing this type of model would allow us to monitor any faults in the overall system and make respective adjustments to have it running smoothly again.
Deep learning
Not only would we utilize this feat of IoT to have this aspect of the textile industry digitized, but there is also potential in using deep learning AI to further facilitate the sorting and distribution processes.
Deep learning artificial intelligence can be implemented into this new system of sorting and overall end-of-life processing for its ease of use, advanced understanding of supply chain networks, and the ability to adapt to changes as systems like this grow and evolve for the better.
Deep learning as opposed to traditional machine learning is more effective at human-like decision-making, which could replace trained professionals over time and make sorting more efficient at facilities like Waste Management. This form of artificial intelligence is highly scalable, whether it be applied to a small sample size like recycling in one town to a commercial enterprise such as Waste Management. Computers would be trained to utilize multiple sets of information (spectroscopic identification, color, etc.) to sort more effectively and enable users to easily identify fiber sources and distribution pathways that products can be sent to for end-of-life processing.
The sorting process would take place at recycling or waste management facilities where the additional process of near-infrared (NIR) spectroscopy can be utilized to identify the specific fiber types that comprise the textile product (https://pubmed.ncbi.nlm.nih.gov/20384118/).
The infrared light, essential to NIR, interacts with the unique functional groups present in different fibers, resulting in a wavelength with distinguishable peaks. The results from NIR along with the visual of the color-coded RFID tags would ultimately give garments and textiles three unique ways of fiber identification for sorting. The addition of NIR along with deep learning AI can be used to assist in the sorting process in the event that digital IDs are damaged or not present on garments or if the color of their tags was faded or removed. It can also open up end-of-life processing to any textile or garment, allowing for this system to be implemented universally.
After the sorting process, distribution of these products to respective recycling facilities, landfills, fuel centers, and even back to their original textile manufacturers could be facilitated with the use of digital twin models and deep learning AI.
The digital twin models would show industries and consumers their products in the real-time pathway toward end-of-life processing. Deep learning AI could then utilize this information to send notifications to consumers to remind them of what steps should be made to ensure that the correct recycling and waste management industries receive and process their products correctly. Ultimately, these technologies and systems would work in tandem and be linked through the advances within the Internet of Things.
Conclusion
Incorporating these various technologies displays just how vast the capabilities of the Internet of Things are and how they could be applied to persisting industries to modernize and make them more environmentally conscious. Together, these technologies drive the industry toward a future where responsible decision-making, real-time insights, and precise material sorting converge, not as contrasting elements, but as a united force steering the textile and fashion industry toward a more sustainable and circular economy.
About the Author
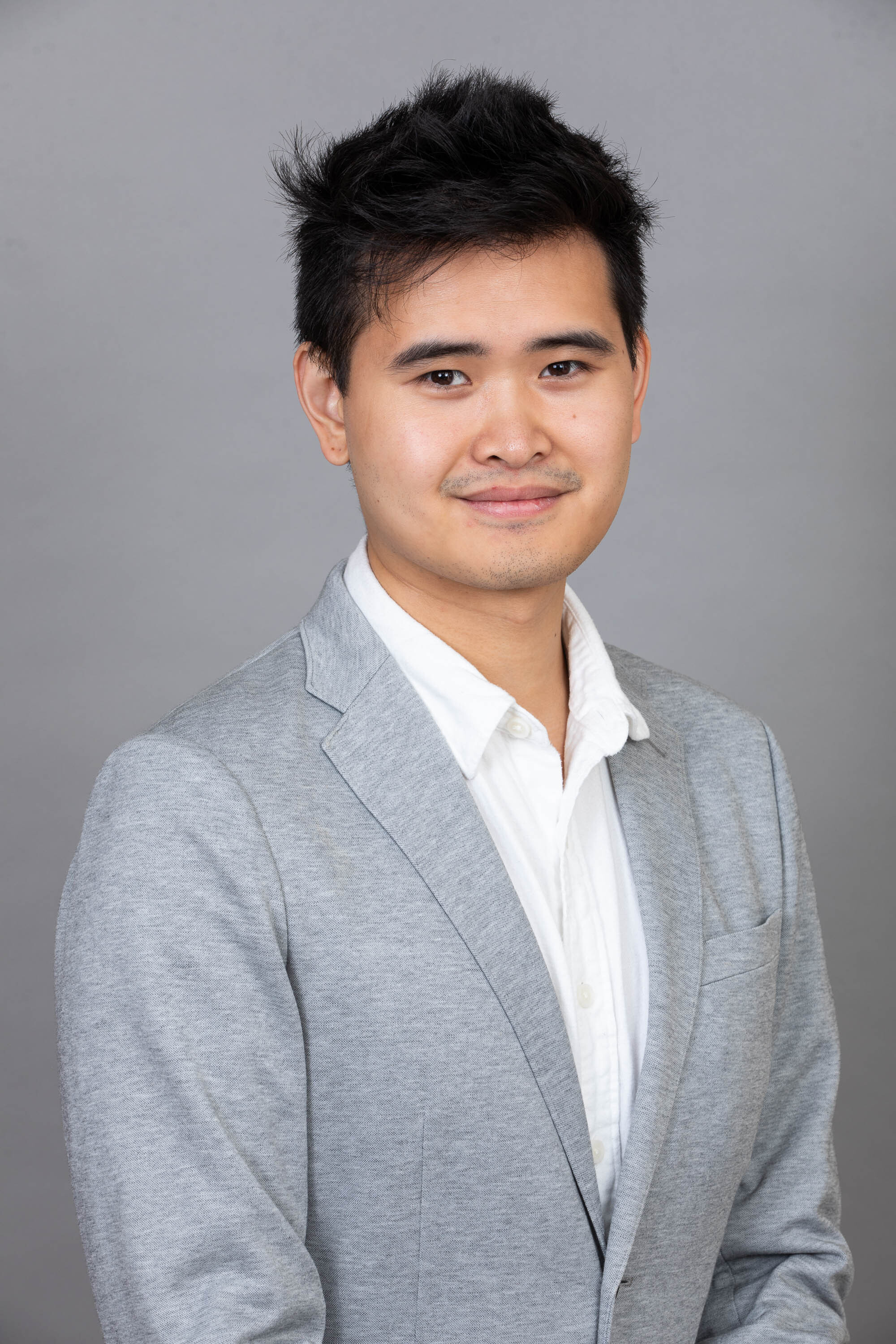
Anderson Ng
Thomas Jefferson University
- Field of Study:Textile Technology
- Expected Year of Graduation:2025
- Chosen Prompt: I chose this topic because I actually have had first hand experience researching and collaborating with industry partners to come up with sustainable solutions with these technologies and systems. Being a textile technology student has opened my eyes to the possibilities of textiles and how such an old institution could be modernized utilizing the innovations of interconnected technology systems.