Renewable energy is our future. Wind and solar are both low-cost, technologically viable, and produce no greenhouse gasses during operation. But renewable power generation has a flaw: it’s inconsistent. Unlike a nuclear or coal plant which can reliably produce a precise quantity of power, renewable sources are at the whim of the sun and the wind. As energy grids must maintain perfect harmony between supply and demand, the non-dispatchable nature of renewable power sources creates a roadblock to the broad implementation of renewable power generation.
Theoretically, energy storage is the perfect solution to this problem. Storing unused energy for later would make it possible to capture wind power in the early morning and then use that power during peak demand in the afternoon. But alas, most current energy storage technology – e.g fuel cells and batteries – is too expensive to be implemented at a large scale. While some storage technology has a relatively low cost – i.e. massive underground caverns to store pressurized air or mountain dams where water can be stored and then run through generators to produce electricity – such solutions usually rely on geographic features which limit the scale of implementation.
As a result, energy storage can only make a minor contribution to supporting a grid, for instance by supporting voltage modulation or providing near-instantaneous power backups. In a world where energy storage is expensive, it is necessary to develop multiple techniques to manage the variability of renewable power production on a grid. First, despite its cost, energy storage at a small scale can be used to help support the instantaneous voltage modulation of the grid. Second, fast response power production, such as black-start natural gas power plants known as ‘peakers’ provide a reliable, but relatively expensive backup when the grid requires extra power. Third, grids can be enlarged to allow for broader access to supply and demand capabilities (e.g. wind farms in Seattle can support the energy demand for air conditioners in Texas). Finally, demand response can be leveraged to trim energy demand during peak hours or increase demand when there is a glut of renewable power. In this paper, I will focus on demand response.
Demand response (DR) presents the practical inverse of energy storage. Rather than attempting to alter the power supply available on the grid, the DR trims or shifts the demand requested from the grid. This requires something conceptually new, but technologically straightforward: the grid operator must be able to communicate directly or indirectly with an energy-consuming device in order to alter its behavior. For example, a dishwasher with demand-side response might be programmed to only run when the cost of electricity is below a certain threshold.
From a technological point of view, DR is much nearer to commercial viability. In order to make the demand-side response a real solution, a grid operator must be able to directly or indirectly communicate with millions of devices, protect against mismanagement from within, and protect against cyberattacks from outside. As a result, demand-side response can benefit mightily from the internet of things, artificial intelligence, and blockchain technologies.
Internet-of-Things
The first limitation of demand response is that renewable energy creates very quick variations (e..g second or minute time scales) in the power supply. If demand is less than supply, energy producers must be curtailed (e.g. disabling a wind turbine) which results in wasted energy. Conversely, if demand is greater, then new power capacity must be quickly brought online (e.g. the peaker plants discussed above), or else a brown or blackout could impact the grid. Automated demand response is a potential solution to this issue of variation, but at the time of writing, much of the U.S. grid is not capable of responding to demand controls at appropriate time scales.
For instance, consider that most consumers in the United States do not have appliances that wirelessly communicate with a grid operator. While the consumers with ‘real-time’ or ‘time-of-use’ pricing can leverage their knowledge of common energy pattens (e.g. energy costs at 5 PM are usually the highest of the day), they would have no realistic method of responding to gluts of wind power which might peak on a time scale of minutes. A viable solution is the implementation of residential appliances which can respond quickly to power pricing information.
For instance, an air conditioner with wireless capability could monitor the real-time cost of power and thus could momentarily pause operation during a price peak or crank up to full operation during energy gluts. Likewise, products throughout the house have the potential to alter their operational characteristics at short and long time scales (e.g. refrigerators, washing machines) in response to such real-time information. The usage of the capability provided by the internet-of-things to produce a nearly instantaneous response to pricing controls results in a power grid’s demand response strategy to fully leverage the value of real-time or time-of-use pricing structures.
Artificial Intelligence
The second problem is an outgrowth of leveraging the internet of things for near-instantaneous alteration of power demand. By creating a system where millions, and potentially billions, of devices on a grid, are individually making decisions about turning on or off, the problem of controlling both producing assets (such as peakers) and power consumers becomes unbelievably complex. While algorithmic control methods may continue to take the lead, the sheer volume of information and quantity of decisions that must be made to support the system’s continued operation is daunting.
A viable pathway to address this challenge of scale is artificial intelligence, or perhaps more accurately for this problem, machine learning. Machine learning techniques are well-suited to help predict trends in the energy grid. For instance, a machine learning model could help predict how a heat wave moving across the United States would impact both renewable power production and rising energy demands and anticipate a potential shortage of power supply.
This prediction, even if available only minutes in advance of rising demand and falling supply, could provide enough warning to allow for the warm-up of so-called ‘peaker’ plants.
Blockchain
The final problem is likewise a corollary to the usage of the internet of things to meet demand response: security against cyberattack. When millions – or possibly billions – of devices must maintain constant direct or indirect communication with a grid operator for the purpose of demand response, cyberattacks can pose a substantial risk and danger to the smooth operation of the power grid and the society at large. To demonstrate the risk potential of cyberattack, if not the full breadth of the relevant risk profile, we can consider the current reliance on the Application Programming Interface or API, which is a ubiquitous component of the internet.
API’s allow internet-capable applications to easily authenticate their identity and relay information. In the case of a demand response strategy instance, a grid operator might create an API that would serve as a centralized location for the many internet-of-things enabled devices to easily connect, authenticate their identity, and then receive power pricing information. Unfortunately, the very convenience of a centralized API creates a bottleneck in the communication process and a weak spot for a distributed denial of service (DDoS) attack.
During such an attack, the targeted API would be inundated with fake queries and be unable to respond to real queries from IoT devices on the grid. The blockchain presents a solution to this problem. Blockchain-based communication protocols do not rely on a single trusted entity, and as a result are partially (though, never say never) immunized against a single point of failure attacks, such as DDOS.
Without a centralized bottleneck, the blockchain provides a practical method of protecting the continued normal operation of a demand response strategy reliant on the Internet of Things.
Conclusion
If a low-cost, large-scale energy storage existed, renewable energy would already be adopted. But alas, no such technology yet exists. Until such low-cost technology is developed, we – humanity – must develop strategies to incorporate renewable energy production through the combination of available technologies. The internet of things will make it possible to develop fast demand response protocols, but will probe the limits of grid monitoring and control systems and exacerbate existing cybersecurity issues. Improving real-time prediction and control for millions of distributed energy assets capable of producing and consuming energy will benefit from the existing development of artificial intelligence techniques. Finally, meeting the cybersecurity challenges will require new thinking about distributed communication protocols, for which blockchain technology will be a valuable asset. These three complementary technologies – internet-of-things, artificial intelligence, and the blockchain – will together help us transition toward a more sustainable society where renewable energy production can meet the majority of our energy needs.
About the Author
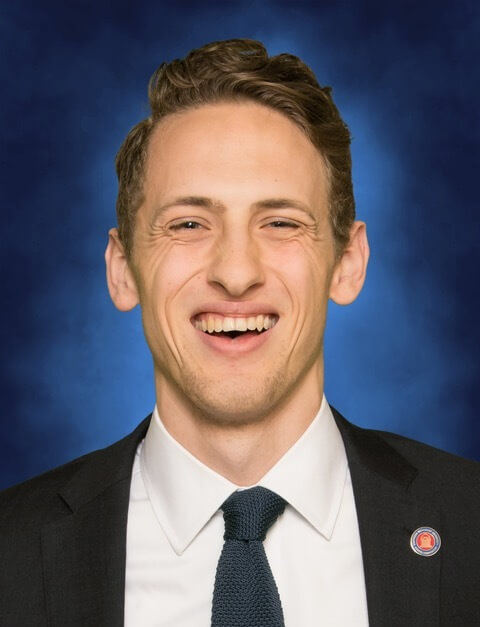
Andrew Timmins
University of Michigan
- Field of Study:Sustainable Energy Systems
- Expected Year of Graduation:2022
- Chosen Prompt: The effect of Blockchain, Artificial Intelligence, and the Internet of Things on renewable energy production and storage.